Exploring The Offshore Indus Basin: Opportunities And Challenges
The Offshore Indus Pak G2-1, the deepest well drilled in the Offshore Indus in terms of water depth, offers interesting insights into the region’s hydrocarbon potential. Despite its depth, the 1D burial history graph (Figure 1) indicates that the well did not achieve the required burial to crack any hydrocarbons. This well, drilled near the Saurashtra Volcanic Arch, encountered a reef, yet remained dry, and the play couldn’t be established.
Reservoir Insights:
Drilling data reveals two sets of proven reservoirs in the Offshore Indus Basin
- Widely Distributed Miocene Channel Sandstones
- Locally Distributed Paleocene–Eocene Reef Limestone
Analogies with the adjacent Kutch Basin and the onshore Indus Basin suggest the possible existence of Cretaceous sandstone reservoirs in the Offshore Indus Basin.
Challenges and Historical Context:
The well stopped in the reef limestone after drilling over 200 meters, and unfortunately, it was dry. It was believed that the adjoining synclines would have generated hydrocarbons that would have migrated to the highs (the reefs) but the concept failed. Since the well was stopped early in the reef, remodeling of data in the context of basin is quite difficult. Had the well penetrated the basement, it would have been quite interesting. It was also believed that the reef would be riding all over the basement rocks. The only proven reservoir is Middle Miocene deltaics that have produced gas in Pak Can-01, but the gas column was too small to justify infrastructure development.
It seems that volcanic activity plays a significant role in the evolution of the offshore part of the Indus Basin and its implications have far-reaching consequences on the hydrocarbon potential.
There have been two major volcanic events in the sea area of Pakistan:
Basalt Eruption of Somnath Ridge (~70 Ma)
Basalt Eruption of Deccan-Reunion (Reunion Mantle Plume, ~65 Ma)
According to Calvès et al. (2010), the basalt eruption of Somnath Ridge contributed to the formation of the volcanic basement in the southeastern Offshore Indus Basin, particularly around Somnath Ridge and Saurashtra High. This area covers approximately 45,000 km².
Geological Insights:
Seismic data interpretation indicates (Figure 2) (that in the southeastern part of the basin adjacent to Somnath Ridge and Saurashtra High, Deccan basalts are distributed in the marine-facies strata of the Upper Cretaceous–Paleocene in a laminated form (Khurram et al., 2019). The northwestern part, far from the Reunion mantle plume, has minimal basalt impact but is close to the strike-slip fault zone of Murray Ridge, making it a potential focus for future oil and gas exploration.
Geothermal Gradients:
Somnath Ridge: Low geothermal gradient of 33℃/km.
Sedimentary Center: High geothermal gradient of 37℃/km – 55℃/km, aiding source rock maturity (Calvès et al., 2010).
The northwestern part, with its developed faults near Murray Ridge, presents an interesting area for future exploration. There are chances that the established Cretaceous plays would be found there (Figure-02).
In most of the wells drilled, the source rocks are in oil window but Pak Can-01 produced gas suggesting that the gas would have been migrated from the deeper part of the basin. Modeling suggests that the Paleocene source rocks (effective in the adjoining onshore) may have become post mature at the end of Oligocene suggesting a charge to the Miocene and younger reservoirs by shallower source rocks.
🔗 Follow us for more insights into the evolving energy landscape and exploration opportunities in the Offshore Indus Basin!
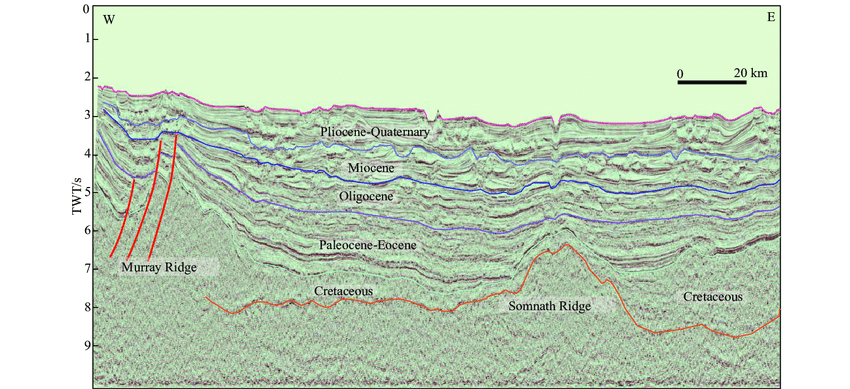
Figure 2 . After Shahzad et. al.
References:
Calves G, Schwab AM, Huuse M, Peter DC, Asif I. 2010. Thermal regime of the northwest Indian rifted margin Comparison with Predictions. Marine and Petroleum Geology, 27, 1133–1147. doi: 10.1016/j.marpetgeo.2010.02.010.
Chatterjee S, Goswami A, Scotese. 2013. The longest voyage: Tectonic, magmatic, and paleoclimatic evolution of the Indian plate during its northward flight from Gondwana to Asia. Gondwana Research, 23, 238–267. doi: 10.1016/j.gr.2012.07.001.
Jian-ming Gonga, b, c, Jing Liaoa, b, *, Jie Lianga, b, Bao-hua Leia, b, Jian-wen Chena, b, Muhammad Khalid, Syed Waseem Haidere, Ming Meng. Exploration prospects of oil and gas in the Northwestern part of the Offshore Indus Basin, Pakistan
Shahzad K, Betzler C, Ahmed N, Qayyum F, Spezzaferri S, Qadir A. 2018. Growth and demise of a Paleogene isolated carbonate platform of the Offshore Indus Basin, Pakistan: Effects of regional and local controlling factors. International Journal of Earth Sciences, 107, 481–504. doi: 10.1007/s00531-017-1504-7.